CHAPTER 5 Recommendations: How to Move to an Operational Analytics Data Architecture Given the benefits cited in the previous chapter, it is obvious that companies need to support a broad set of analytic requirements for IoT applications, including: 1. High-performance transactional processing 2. Streaming analytics s tic aly an al n tio a er p o 3. Real-time insights t h g ri he e h T es all t e mak r u ect chit 4. Machine learning ar ailable in one v a a t needed da or: e f lac p s tic By selecting the right architecture—one that plugs into an existing aly an s es n si bu - f -o e n Li such benefits can be realized. infrastructure with minimal disruption— s t h g si n i e tiv u ec x E g tin An ideal solution would be able to perform all workloads within a r o ep R e ernanc v o single database. This would allow an organization to store and e & g lianc omp C ses u t ha process data in a single, operational analytics processing platform, e t a scienc t a D enabling synergies across operational areas. Additionally, the right AI and solution can help deliver real-time insights with high availability to ML data 24x7, and low-latency access to the data. Moreover, a suitable operational analytics processing solution must avoid errors and problems that occur in other analysis systems where data must be extracted, transformed, and loaded (ETL) before it is analyzed. The right solution overcomes these problems by delivering a real-time, trusted view of critical data. This ensures that information is accurate and helps guarantee that the same data is used across the organization. An additional issue to consider when selecting an operational analytics processing solution is the ability to run transactional and analytic workloads at the scale required by today’s industrial IoT efforts. A Modern Analytics Platform New processing requirements and analysis workflows need a platform that can easily ramp up capacity as data volumes and streaming speeds increase. Legacy relational databases can’t be scaled easily. Limitations are so severe that transaction processing is run as a batch process, with the database unavailable or degraded for queries during processing. A complex ETL process must then be run to ready data for analytics; and analytics, even against this stale data, are often not available in real time. The need for 24x7 data collection and monitoring can generate very large tables, resulting in slow-perform- ing queries. A common trade-off is to break up the data into separate silos or batch the data into low-cost data lakes—which requires the use of caching, an additional layer that demands expert, ongoing tuning. The manual work involved leads to query delays, inaccurate results that can ultimately cause customer attrition, and increased costs for performance workarounds. NoSQL databases overcome the scalability barrier but are designed for unstructured or semi-structured data. So, they can’t natively maintain consistency and proper join patterns for a structured data store, as required for efficient analytics. This makes NoSQL less than ideal to fully address the problems of legacy operational data for real-time or near-real-time decision-making at scale. Copyright (©) 2019 RTInsights Industrial IoT Data Collection & Analysis for Real-Time Decision-Making and Predictive Maintenance 11
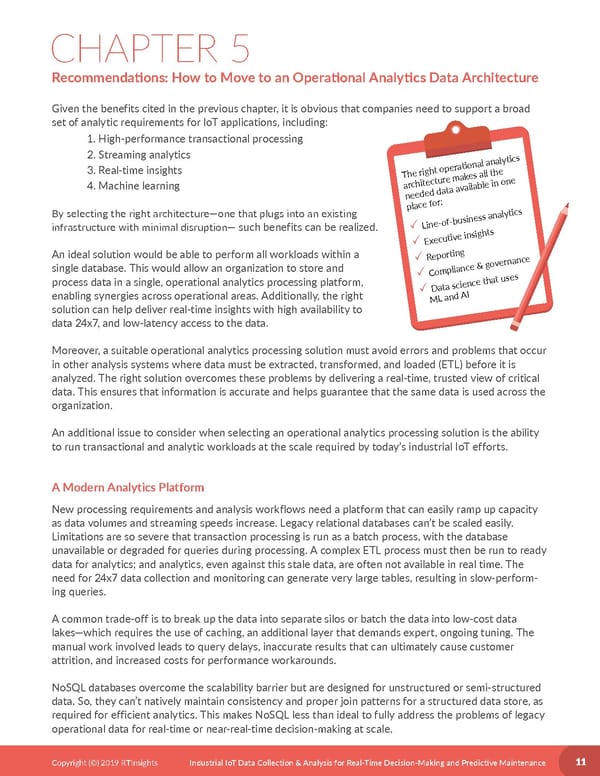