Another factor to consider in the architectural choice is performance. An organization might have data coming from many thousands of IoT sensors and monitors. The platform must be capable of high- performance computing and sustained workflow throughput to enable analysis of large volumes of real-time data on the fly. Given the business-critical use of the information derived from such an analysis, the solution selected must deliver enterprise-class reliability, security, tools, and features. Taking these requirements together, the critical elements in an operational analytics architecture include: · A core data platform that supports high availability, security, performance, scalability, and management · Data integrity and consistency to deliver trusted data · Native tools or integration with third-party vendors’ tools to support data management functions · Support for concurrent queries, transactions, reports, and data access patterns · Deployment options for on-premises or cloud · Access to data using standard connectivity such as SQL, ODBC/JDBC, XML, or REST Enabling New Types of Analysis A solution that incorporates the above critical elements would be able to perform the analysis of IoT data needed in industries today. The information derived in such an analysis could, for example, take predictive maintenance in new directions. For example, a common way to avoid equipment downtime is to replace a component when it gets close to its Mean Time to Failure (MTTF) age. There are two issues with using such an approach. A part may fail before its MTTF age, leading to unplanned downtime. Or, a part that is fully functioning may be replaced well before it actually fails, thus making less than the maximum use of that part. ML-based analytics run on streaming IoT data and complemented by product history information could be used to spot precursors to trouble in a part that has not reached its MTTF age. Similarly, that analysis could be used to keep a part in service for an extended period of time, knowing the analysis will tip off service staff before the failure occurs. Copyright (©) 2019 RTInsights Industrial IoT Data Collection & Analysis for Real-Time Decision-Making and Predictive Maintenance 8
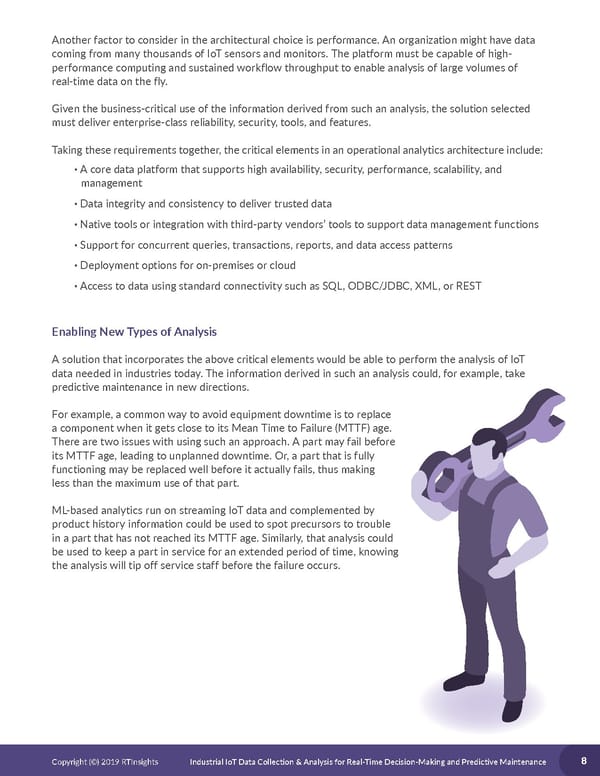