Making use of, and efficiently managing and analyzing such large volumes of data, which are continu- ously generated, requires new thinking. Many companies are complementing traditional analysis with real-time, artificial intelligence (AI) and machine learning (ML) algorithms to get decision-making infor- mation out of the data in a timeframe that will allow a company to take proactive actions. Specifically, industrial uses of AI and ML applied to IoT data are seen as a great way to supercharge and automate maintenance forecasting and other aspects of operations. The reason: AI and ML can more accurately and quickly help automate insights and decisions about complex systems. Using these more sophisticated analysis techniques, predictive maintenance can reduce machine downtime, thus lowering costs and improving margins. Areas where AI and ML could help industrial operations include: Equipment uptime and availability: Telemetrics about the state of a device or machine combined with historical performance data can provide insights that foreshadow preventable failures. Quality assurance (QA): Better optimized and automated QA processes not only reduce costs but also enhance product quality. Assembly line optimization: IoT data can be used to identify inefficiencies which, once addressed, can increase output. Other ML use cases that can help industrial operations include supply and demand forecasting, data security, and supply chain logistics and management. Copyright (©) 2019 RTInsights Industrial IoT Data Collection & Analysis for Real-Time Decision-Making and Predictive Maintenance 4
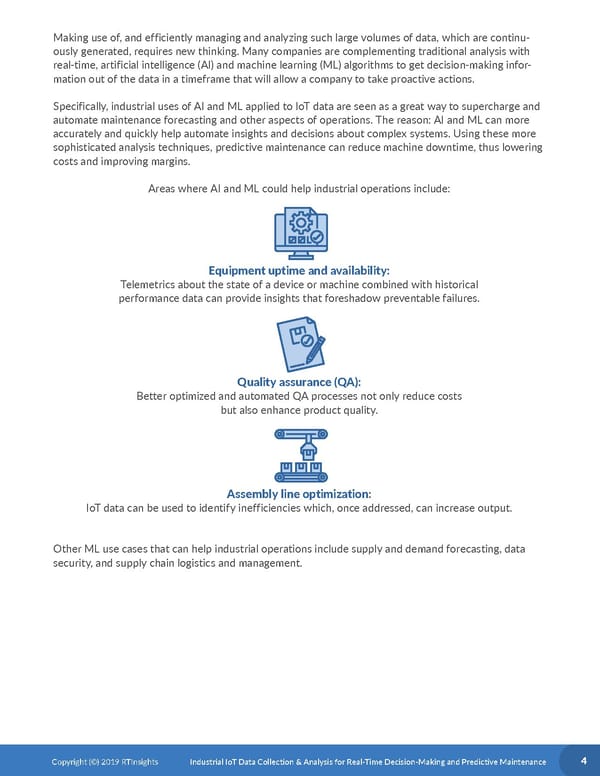