CHAPTER 2 Barriers to Success The combination of large volumes of streaming data coming from IoT devices, plus the use of sophisti- cated AI and ML models, creates the need for a new kind of data infrastructure that combines opera- tional analytics with new data processing techniques to sense problems and respond to them before issues arise. The idea of using IoT devices to track machine readiness and automate predictive maintenance in industrial applications is widely appealing. However, most organizations do not have the data infrastruc- ture required to take full advantage of these potential benefits. And there are many challenges that must be overcome to achieve success. Identifying the Challenges Most data scientists spend only First, there is the issue of data preparation. To harness the value of 20% IoT and deliver innovation-driving insights, organizations must quickly of their time prepare and standardize great volumes of disparate, unstructured data. on data analysis. In the industrial world, large industrial organizations can typically collect billions of data sets from machines, sensors, and internal business applications. Data preparation takes up to 80 percent of the time and resources in a data analysis operation.2 As organizations move to new IoT initiatives, it’s important to consider new technologies and processes that will keep up with this huge influx of data. Another issue is data complexity. Many IoT devices may generate data with timestamps or geotagged data. But this data must be combined with other structured sources to be of use. The complexity is only multiplied as more data is generated. Common data preparation tools like Excel can’t handle this complexity, which leaves skilled analysts locked out of working with the data. Data integration is yet another obstacle to success. Most analysis infrastructures are not designed to exchange or process the vast amounts of complex information pulled from sensors and connected 80% devices. It’s difficult to quickly integrate and enrich machine-generated data with data from business applications and data repositories. Greatly of their time adding to this problem is the fact that much of the data is siloed. is spent finding, cleaning, and reorganizing data. 2 https://www.infoworld.com/article/3228245/the-80-20-data-science-dilemma.html Copyright (©) 2019 RTInsights Industrial IoT Data Collection & Analysis for Real-Time Decision-Making and Predictive Maintenance 5 Copyright (©) 2019 RTInsights Industrial IoT Data Collection & Analysis for Real-Time Decision-Making and Predictive Maintenance
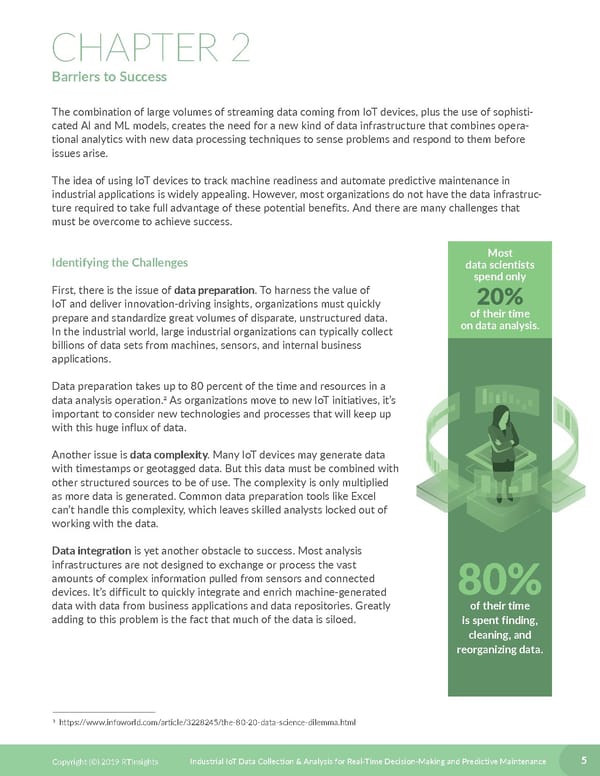