Traditional analytics processing would first collect and store multiple streams of data and then put that data into a relational format for real-time or historical analysis, which is commonly executed using slow batch processing. These tasks can stress traditional analytics infrastructures, resulting in significant analysis delays or an inability to run workloads. This precludes the instant discovery of anomalies and rapid generation of predictions. Scalable stream ingest also must be addressed since most traditional systems either struggle to collect every event, or store data in an inefficient and costly way. Users are then forced to do all sorts of tricks to move or delete data onto less costly storage media. Naturally, usability can be an obstacle to success with any operational analytics endeavor. Many times, a solution will be used that requires deep data science expertise to craft queries and conduct investiga- tions. This greatly limits the use of sophisticated analytics within the organization and incurs delays as business and operational staff wait for the data scientists to perform their duties. Finally, there is the issue of concurrency. In the rush to solve for the challenges of the volumes of IoT data and large numbers of concurrent users, some solutions abandoned the core features of databases that make them highly performant and easy to use. Adopting New Approaches to Analytics Simply put, these challenges are too much for current infrastructures. They cannot perform the needed operations to deliver decision-making intelligence in a timely enough manner. Not only does a great amount of data need to be analyzed, but the true value also comes from a system that can continuously monitor, identify, and respond to opportunities or issues in real time. This situation is leading organizations to adopt new approaches to their traditional analytics efforts. Specifically, organizations are looking to leverage the power of AI and ML to spot critical information in real-time data. This is causing operational strains as siloed data and slow analytics prevent the power of AI and ML from being brought to bear. In response, organizations are moving to operational analytics. This is a hybrid processing approach that depends on the ability to run both transactional and analytical workloads at scale on an integrated SQL database. The ability to run both kinds of workloads on a single platform has been given a variety of names, from hybrid transaction/analytical processing (HTAP), to hybrid operational- analytics processing (HOAP), or translytical data platforms. In this genre of analytics, in-memory processing is a key technology enabler. It delivers the performance needed to analyze streaming data. An added benefit is the simplicity of the architecture. Only one system needs to be maintained, and there is no movement of data. Copyright (©) 2019 RTInsights Industrial IoT Data Collection & Analysis for Real-Time Decision-Making and Predictive Maintenance 6
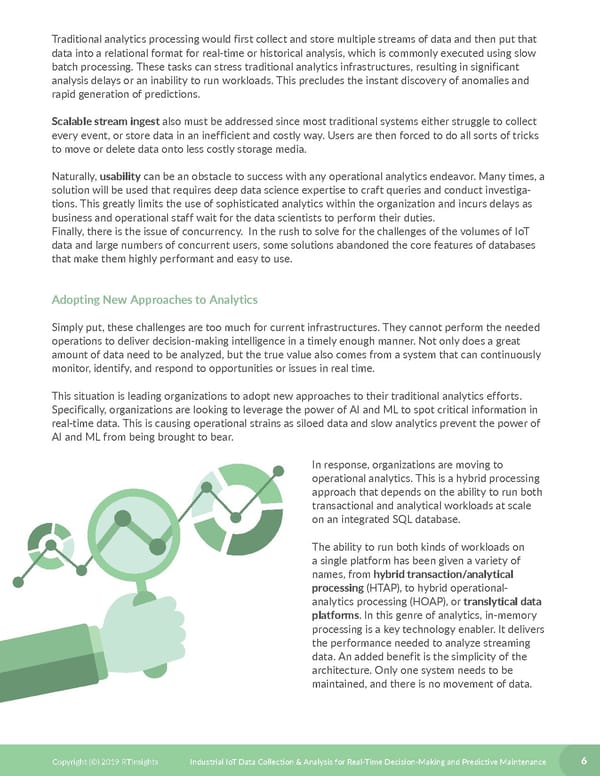